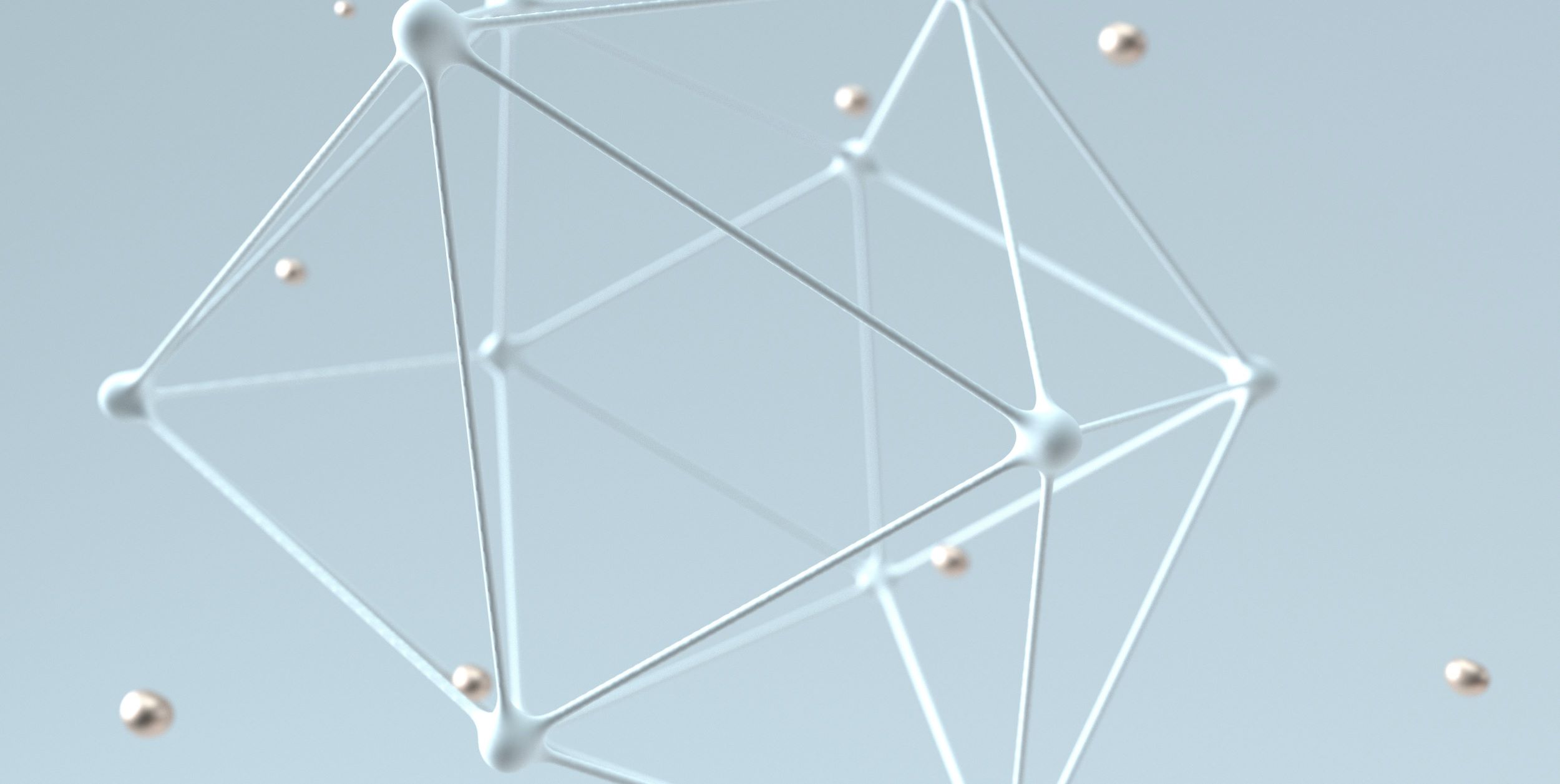
AI/ML Challenges in Pharma Research & Development
Please help us identify and prioritize pre-competitive AI/ML challenges and opportunities in Pharma R&D.
Please help us identify and prioritize pre-competitive AI/ML challenges and opportunities in Pharma R&D.
Life Science Professionals say Agentic AI to Have Most Impact in Next 2-3 Years, but 51% Cite Resistance to Change as Biggest Barrier to Innovation
New initiative will collect pharma and CRO data to refine industry carbon measurement of technologies
We are honoured to have won a 2025 Bio-IT Innovative Practices Award for our FAIR Maturity Matrix and an Honourable Mention for our In Vitro Pharmacology (IVP) project.
The award, which honours rising stars in data science, received entrants from across the global biopharmaceutical ecosystem and competition was fierce.
Pistoia Alliance launches 2025 Lab of the Future survey in collaboration with Open Pharma Research.
This inaugural industry award has been established to recognise, nurture and reward emerging talent in life sciences.
The Pistoia Alliance has announced the results of a global survey in conjunction with CCC on the impact of AI and copyright legislation on pharma R&D.
The Pistoia Alliance has launched a new industry benchmark report: Accelerating Digital Transformation in Pharma with IDMP.
Collaborative white paper highlights misaligned incentives in R&D are holding back full human data integration and better patient outcomes.
As a member of our community, take the opportunity to contribute to our annual global Lab of the Future survey 2024